What is multi-touch attribution marketing?
Multi-touch attribution (MTA) is the practice of assigning credit to multiple marketing touchpoints in proportion to their impact in driving a desired conversion outcome. The operative term here is “proportion.” Multi-touch attribution models collect data from all touches in the buyer’s journey and assign proportionate credit based on the attribution weightings of a model, thereby accurately reflecting their impact (or not) in generating a sale or a specified conversion event.
For example, if a prospect or visitor comes to your website through a search ad, converts to a lead on a newsletter, engages in person with a sales person, and revisits your website through a remarketing ad to make a purchase for $100, multi-touch attribution (using a linear attribution model for illustrative purposes) would assign $25 in proportionate revenue credit to each of the four touches. And depending on your view of the impact of the recency of the touch, multi-touch attribution models come in different models to easily fit your strategy.
Unlike multi-channel attribution, multi-touch attribution can track any marketing touch with a deep level of granularity, such as online channels (any source, medium, campaign, etc.), events (any sales touch), email campaigns, and even direct mail, mobile devices and television.
What problem does multi-touch attribution marketing solve?
Remember the earlier word, “proportionate”? The problem is single-touch attribution allocates a disproportionate amount of revenue credit to either the first or last touchpoint. If your customers’ purchase path is straightforward, such as ecommerce attribution often is, then single touch attribution is an adequate method of informing marketing budget allocation using tools like Google Analytics and your CRM. However, like most modern B2B and B2C businesses, you’re probably marketing through multiple paid platforms and marketing channels including offline, single-touch attribution misrepresents ROI by giving 100% revenue credit to either the first touchpoint or last, woefully understating the impact of other touches and channels.
Further, single-touch attribution data from ad-specific ad sources such as Google Analytics and Facebook Insights often double-count revenue with each source claiming 100% revenue credit to a sale. Other approaches like Marketing Mix Modeling have their own flaws: providers often don’t show you the P-score so you don’t know how accurate the insights are. With this “attribution anarchy,” marketers can’t make data-informed decisions as to which channels and campaigns are worth spending more dollars on and which ones are wasting dollars.
What are the different multi-touch attribution models? Which one is right for my marketing?
While single-touch attribution only gives credit to one marketing touchpoint, multi-touch attribution models assume all different touchpoints play some role in driving a conversion such as a lead, pipeline or a sale. Here we’ll look at the most popular multi-touch attribution models and how you can choose the one that will give you the deepest insight into revenue and lead attribution.
Linear multi-touch attribution model
A linear or “impartial” model is the baseline for multi-touch attribution models. It assigns an equal percentage of revenue credit to each touch regardless of its recency in the buying journey. Hence, using the earlier example, each touchpoint receives an equal $15 in revenue credit, totaling $100.
If you don’t have a strong view of the value of when the touch took place in the buying journey, then a linear model is an excellent starting point. Don’t try to boil the ocean! The good news is with a modern multi-touch attribution tool you can easily toggle between attribution models to see the differences and start learning.
Time-decay multi-touch attribution model
A time-decay model assigns revenue credit to each touch based in accordance to its recency in the buying journey. Whereas a linear model gives equal credit, a time-decay multi-touch attribution model says the closer the touch was to the sale or conversion event, the more influential it was. Using the same buyer journey, you can see the more recent touches receive more credit than the older ones.
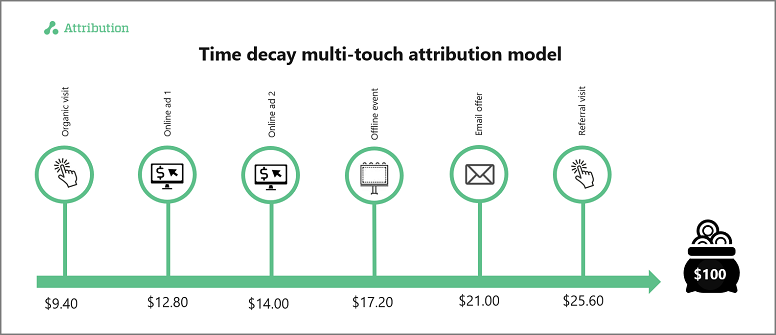
If you believe strongly (and have some supporting data) that more credit should be allocated to touches that nudged prospect closer to a sale vs touches that brought the lead or buyer in, then a time-decay multi-touch attribution model is the way to go.
Position-based (or U or W-shaped) multi-touch attribution model
A position-based (or U or W-shaped) model assigns 40% revenue credit to the first and last touch prior to the conversion event or sale, with the remaining 20% distributed equally among the middle touches. If there is only one touch before a conversion event or sale, then a position-based multi-touch attribution model would give 100% revenue credit to the touch. Similarly, if there is only a first and last touch, they each receive 50%.
Let’s look at our example to see how these models work:
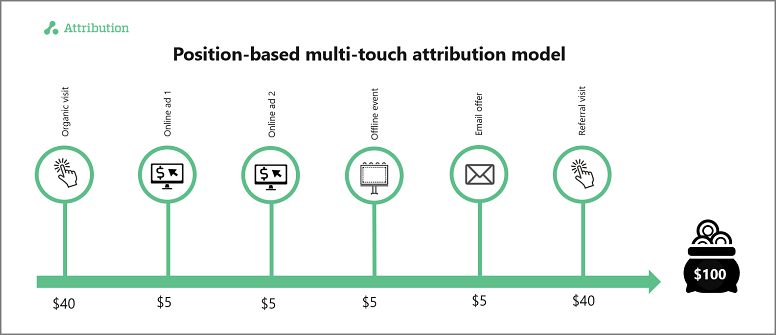
Note: A W-shaped model works the same as a U-shaped attribution model except it allocates 30% revenue credit each to the first, key middle, and last touch, while distributing equally the remaining 10% among other middle touches.
If your business places more value on the touch that brought in the initial prospect and the touch that converted them compared to the middle of the buyer journey touches, then you should use a position-based multi-touch attribution model to optimize your campaigns and channels.
Data-driven machine learning multi-touch attribution model
Custom machine learning modeling is the most advanced approach to multi-touch attribution. A custom attribution model uses a machine learning algorithm to assign revenue credits to touches. Rather than using a static model with user-determined weightings as in the above examples, an algorithm is derived using historical touch and conversion data. As you can see in the example below, data-driven attribution modeling applies a unique algorithm to allocate credits.
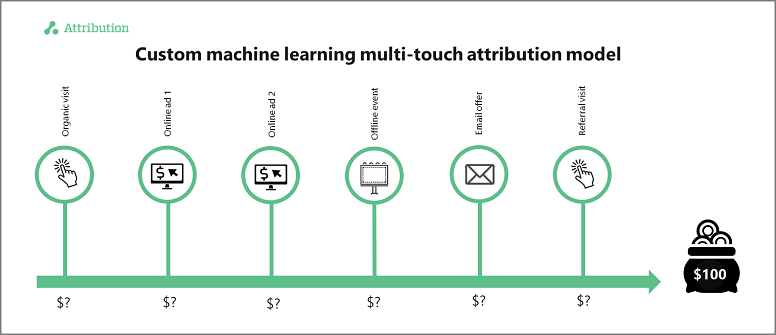
It is for this reason you should not expend the effort with it until after you’ve gone through at least one or two full path buying cycles using one or more of the static models. If you’re business is direct-to-consumer with a short purchase path, this means a matter of a few weeks, buy if you’re dealing with longer B2B buyer journeys I would learn from the static models for a good six or more months depending on your sales cycle to determine if there is ample opportunity to further optimize your marketing mix.
There’s no perfect science for choosing the right multi-touch attribution model. As the use of multi-touch attribution to optimize budgets and channels matures, marketers will run several models at one time to see which one is right for their business. The good news is modern multi-touch attribution tools allow you to see and compare the results from each model.
5 signs that your company is ready for multi-touch attribution
Regardless of whether you’re a B2B or B2C marketer, multi-touch attribution is worth the effort if you’re on the hook for lead or pipeline generation and/or customer acquisition or purchase, AND you’re:
1. Using multiple digital ad networks
Many companies are using various digital ad networks such as Google Ads, LinkedIn Ads, AdRoll, Facebook Ads, and others. As the number of ad channels grow, single-touch attribution crumbles under the tracking complexity. With less linear and more complicated customer purchase paths, multi-touch attribution effectively manages the attribution intricacies of different ad channels (and the products and metrics within each channel required) for informing budget and revenue allocation across the different ad platforms.
2. Using or considering using offline channels
Common offline channels include direct mail, conferences, stores and/or sales touches. The minute you expand beyond digital marketing and do offline marketing is the moment single-touch marketing attribution tools such as Google Analytics become useless in giving you holistic and accurate visibility of the ROI of your online and offline channels. Multi-touch attribution is channel-neutral, thereby allowing you to accurately compare ‘apples to apples’ ROI between online advertising and event spending, as well as the insight within each offline channel.
3. Using or considering using SEO to spur brand awareness and qualified traffic to your site
Here only multi-touch attribution can connect anonymous touches from your blogs and web pages, and accurately attribute revenue and cost using the same model (and conversion data) as your other channels.
4. Spending more than $250,000 annually to drive B2C sales or $500,000 annually to generate qualified B2B leads or pipeline
This is considered a general inflection point where your marketing efforts are increasingly better spent on knowing which channels and campaigns are working and which ones need to be purged for poor ROI vs getting mired in attribution chaos due to lack of true attribution insight.
5. Trying to optimize budgets using single-touch attribution from one or more tools
If you have a short and simple marketing funnel, a single-touch model (e.g., first-touch attribution or last-touch attribution) may be good enough. But if you’re marketing on various channels, have many touch points and are nearing “attribution anarchy” with single-touch tracking, you’re ready to move to multi-touch attribution marketing to augment your decision-making. Now.
Multi-touch attribution marketing is both a technology and culture
Because buyer journeys span many touchpoints and devices before converting, marketers need to understand which touchpoints a buyer (or buyers in the case of B2B account-based marketing) interacted with that resulted in a positive action. The goal is to understand where to focus spend, devoting funds to higher performing channels and campaigns and diverting dollars from those that were ineffective, making the change to multi-touch attribution marketing is as much a culture shift as it is a new technology.
With the click of a mouse, it enables marketers to look at all channels and attribute the sale to those touches using various models. Marketers can look at user-level data (clicks, forms) their touches have on key conversion goals such as leads, signups and purchases. Unlike single-touch attribution, multi-touch attribution models enable marketers to better understand both the chronology and the type of interactions that preceded and influenced conversions and accurately apportion conversion credit to touchpoints, using the insight to optimize the conversion paths of buyers.